Causal Inference vs. Correlation: Why Data Science Needs a Paradigm Shift
- Brinda executivepanda
- 1 day ago
- 2 min read
In data science, we often hear the phrase “correlation is not causation.” Yet, many decisions are still made based on patterns that may not reflect true cause-and-effect relationships. As businesses rely more on data to guide strategies, it’s becoming clear that a shift toward causal inference is necessary for more reliable and meaningful results.
Why Correlation Isn’t Enough
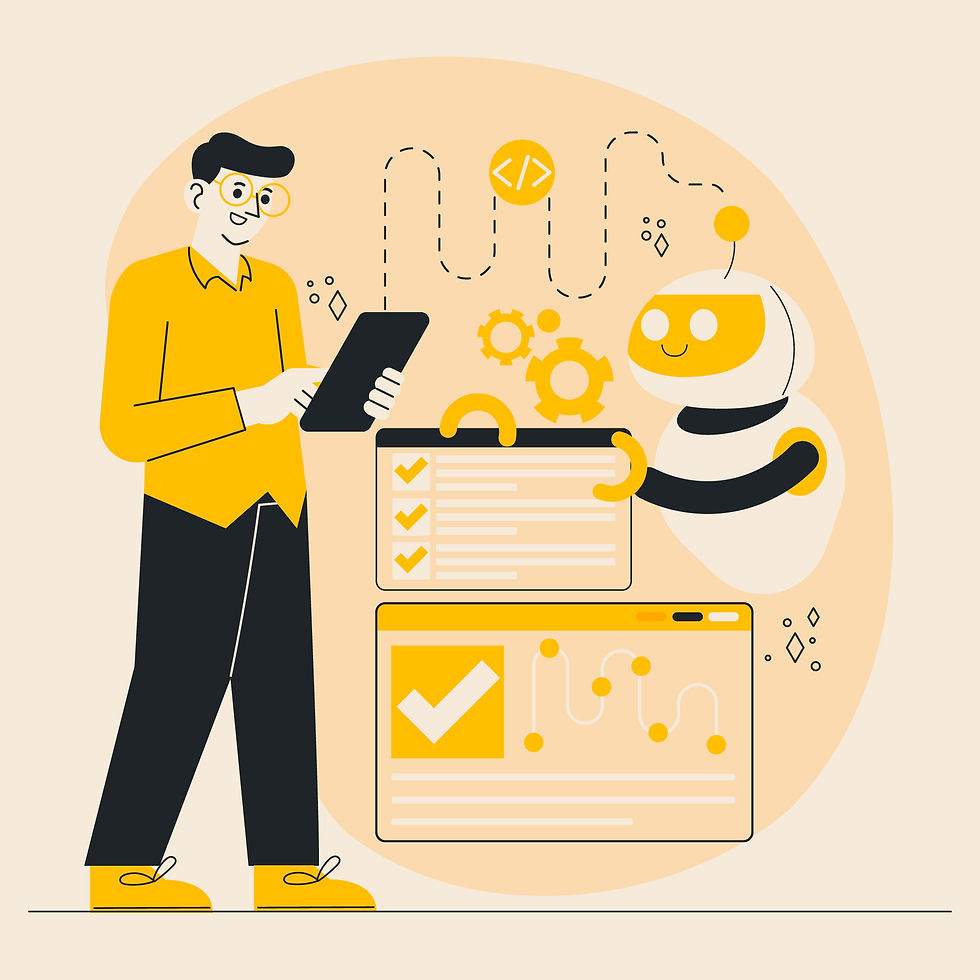
Correlation tells us when two things move together, but it doesn’t explain why. For example, ice cream sales and drowning incidents may rise in the summer, but one doesn’t cause the other. Relying only on correlation can lead to wrong conclusions, which can affect important decisions.
The Power of Causal Inference
Causal inference focuses on understanding the impact of one factor on another. Instead of just seeing patterns, it helps us ask, “What would happen if we changed something?” This approach is more useful for making changes, testing strategies, and improving outcomes.
Real-World Applications
Causal models are used in healthcare to find out if a treatment really works, in marketing to measure the true impact of a campaign, and in policy-making to test the effects of new laws. These are decisions where guessing wrong could have serious consequences.
Challenges in Moving to Causal Thinking
Causal inference needs careful planning, good data, and often experimental or quasi-experimental setups. It’s harder than running a quick analysis, but the results are more trustworthy. Data scientists also need the right tools and training to use this approach well.
Comentários